Artificial intelligence (AI) has taken over the internet within the last year. What’s more, AI has emerged as a game-changer for businesses across the globe. The integration of artificial intelligence in business operations is not just a trend but a pivotal strategy for companies seeking to gain a competitive edge. As a leader in the realm of business process automation (BPA), Exela is furthering our commitment to investing in AI and Data Science. This move underscores our dedication to staying at the forefront of innovation and offering cutting-edge technology solutions to our clients. In this post, we'll explore the key aspects of implementing AI in your business operations to help you navigate this transformative era.
Understanding AI and Its Business Implications
AI represents a sophisticated blend of algorithms and computational power designed to think, learn, and act – a simulation of human intelligence in machines. The potential of artificial intelligence in business involves extracting actionable insights, automating complex processes, and continuously learning from interactions and outcomes. This technology is reshaping industries by personalizing customer experiences, optimizing supply chains, and even predicting market trends. AI's ability to rapidly process and learn from data can significantly enhance operational efficiency, cut costs, and foster innovation, making it an essential component for businesses aiming to thrive in an increasingly digital world. Its application spans various domains – from advanced customer service chatbots and predictive maintenance in manufacturing to intelligent analytics for strategic decision-making – demonstrating its versatility and transformative impact.
AI presents many possibilities from chatbots, to data extraction, to research assistance, and the list goes on and on. With so many options, leaders may be confused about which AI tools to implement into their business. If this sounds familiar, read on!
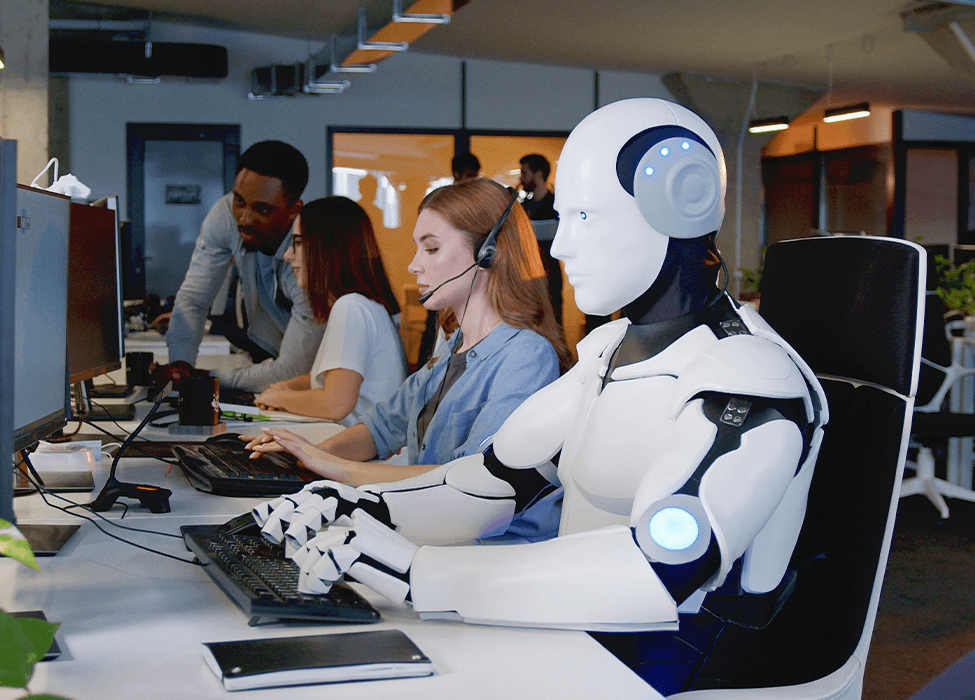
Strategies for Implementing AI in Your Business
Identifying the Right Opportunities: Begin by assessing areas within your operations that can benefit the most from AI. This might include customer service, data analysis, inventory management, or financial operations. The key is to identify processes that AI can optimize for efficiency and effectiveness. Pay particular attention to operations where employees may be struggling with workload or inefficient, slow, or outdated processes.
Choosing the Right AI Technology: Most high-impact AI technology today is Generative AI leveraging LLMs (Large Language Models). Other AI technologies, such as machine learning (ML), natural language processing, and robotics, have a high impact on businesses as well. Each serves different purposes and it's crucial to choose the technology that aligns with your specific business needs and goals.
Here are the areas that have the highest possible business impact when you adopt Generative AI with LLMs.
- - Customer Services
- - Sales and Marketing
- - Product R &D
- - Supply Chain
- - Software Engineering
Most of the state-of-the-art Gen AI models like OpenAI, Google Gemini, Meta LLama2 and a host of open source models built by companies at the cutting edge of AI provide the right starting point in building AI applications.
Imagine an online retail company that wants to enhance its customer experience and streamline its operations. Here's how it might select appropriate AI technologies:
Customer Service Enhancement
- - Business Need: Improving customer support with a 24/7 available, efficient, and responsive service.
- - AI Technology: Natural Language Processing (NLP).
- - Application: Implementing an AI-powered chatbot that uses NLP to understand and respond to customer queries in natural language. This chatbot can handle a wide range of customer service inquiries, from tracking orders to handling returns, thereby improving response times and customer satisfaction.
-
Inventory Management
- - Business Need: Optimizing inventory levels to reduce storage costs and avoid stockouts.
- - AI Technology: Machine Learning (ML)
- - Application: Using ML algorithms to analyze sales data, predict future demand patterns, and optimize inventory replenishment. This AI-driven approach helps the company maintain just-in-time (JIT) inventory and manage third-party logistics and supply chain effectively.
-
Warehouse Operations
- - Business Need: Streamlining warehouse operations for quicker and more accurate order fulfillment.
- - AI Technology: AGV (automated guided vehicle) / AMR (autonomous mobile robot)
- - Application: Deploying robots in the warehouse to assist with picking and packing orders. These robots can navigate the warehouse efficiently, retrieve items quickly, and reduce errors in the fulfillment process, thus speeding up the overall order processing time.
-
In each of these cases, the chosen AI technology aligns with a specific operational need of the online retail company. By carefully selecting AI technologies that match its unique business requirements, the company can effectively leverage AI to achieve its goals and enhance its competitive advantage in the market.
Data Management: AI systems require data to learn and make decisions. Ensure you have a robust data management system in place. This involves collecting high-quality, relevant data and maintaining a secure and scalable data infrastructure.
Talent and Training: Investing in the right talent is critical. This may mean hiring new staff with AI expertise or training your existing workforce. It’s important to foster a culture of continuous learning and adaptation.
Ethical Considerations and Compliance: Implement AI in a way that is ethically responsible and compliant with industry regulations. This includes addressing issues like data privacy, detecting bias in AI algorithms, and providing transparency in AI-driven decisions. We’ll talk more about the limitations of AI in the next section.
Addressing the Limitations and Risks of AI
AI systems, at their core, are dependent on the data they are trained on, making them susceptible to biases and inaccuracies if the data is flawed. This limitation underscores the need for human oversight in AI-driven processes to help ensure fairness, ethical considerations, and accuracy.
Security and privacy concerns are heightened with AI, as these systems often handle sensitive data, making robust cybersecurity measures essential. Thus, while AI is an invaluable tool for efficiency and growth, it should be complemented with human expertise and critical thinking to help ensure balanced and effective business operations.
Recognizing these challenges and the need for a balanced approach in AI adoption, many businesses are turning towards strategic solutions that blend the best of human expertise with AI's capabilities. One such effective strategy is partnering with an AI enablement firm who has already walked this path. This approach augments business-aware in-house teams to enable AI solutions addressing specific problems instead of a generic AI solution approach.
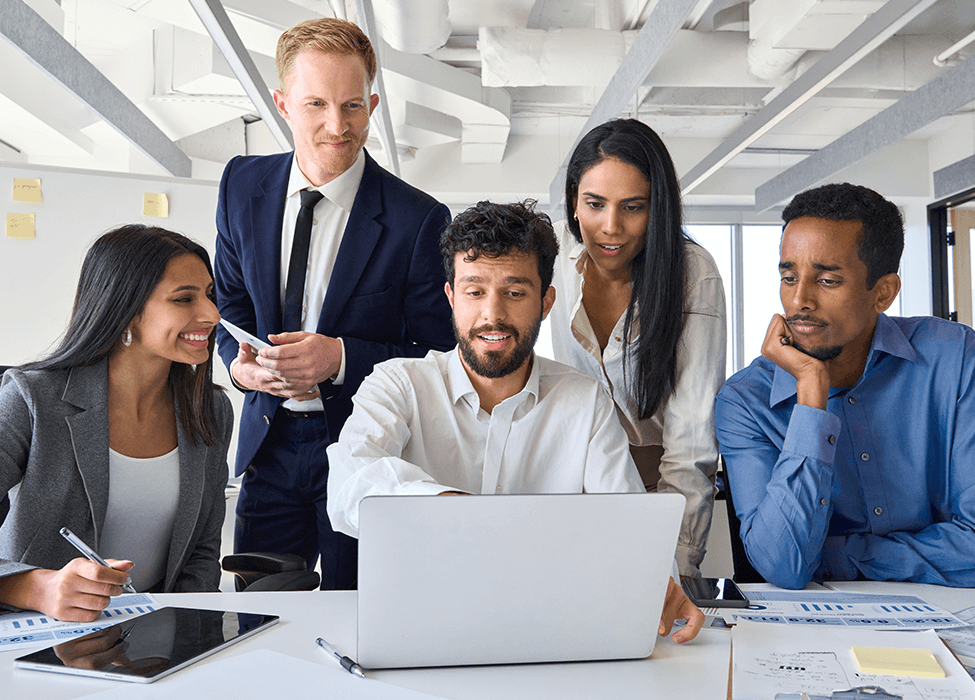
Leveraging AI Experts
An increasing number of companies are recognizing the value of outsourcing to harness the power of artificial intelligence in business. Partnering with AI experts allows businesses to tap into a pool of expert talent and advanced technologies without the need for heavy investment in in-house development. This approach is particularly beneficial for small to medium-sized businesses that may not have the resources to fully develop their own AI capabilities. By using an AI-enablement firm, companies can quickly implement AI solutions, scale their operations, and stay competitive in their industry.
Additionally, this strategy mitigates the risk and complexity associated with AI adoption, as external experts manage the nuances of development, implementation, and maintenance. Whether it’s for data analysis, customer service automation, or predictive analytics, outsourcing AI operations can be a strategic move to optimize business processes and drive innovation.
Reaktr.ai: Your Partner in AI-Driven Business Transformation
In an era where artificial intelligence is pivotal in business transformation, Exela’s Reaktr.ai emerges as a key provider, offering a comprehensive suite of AI-driven solutions. Reaktr.ai is engineered to simplify the technological complexities faced by businesses, enhancing operational agility and precision through its diverse AI capabilities.
Brief Overview of Reaktr.ai's AI Solutions
Data Modernization
Reaktr.ai efficiently addresses the challenges of utilizing private data for AI in business, focusing on data irregularities and adapting to new technologies. It conducts thorough data source inventories and quality assessments, targeting up to 98% data accuracy and faster AI implementation cycles. Its AI-infused platform manages data lifecycle for accuracy, security, and compliance, in the effort of significantly reducing costs. Additionally, Reaktr.ai provides customized AI models and maintenance services, streamlining the integration of AI tools and reducing development time and costs, thereby equipping businesses to effectively navigate the AI technology landscape.
AI & ML
Reaktr.ai offers a cutting-edge Early Warning Bot that serves as a vigilant monitor in the digital landscape, tracking over 1000 data parameters across users and devices for operational stability. This tool, combined with our advanced fraud detection system using generative AI and language models, significantly enhances transaction security by reducing false positives and improving fraud detection accuracy.
Additionally, Reaktr.ai's Generative AI capabilities extend to predicting network anomalies in complex telecom systems, leading to reduced network downtime and customer complaints. Together, these AI-driven solutions by Reaktr.ai represent a comprehensive approach to enhancing operational security and efficiency in a dynamic digital environment.
Multi-Cloud Management
Reaktr.ai’s CloudX streamlines multi-cloud management, making it cost-effective and efficient. This service simplifies the full life cycle of multi-cloud operations, from setup to maintenance, and is available both as a service and through a user-friendly SaaS platform. CloudX can significantly cut cloud costs by up to 30%, reduce operational expenses by up to 25%, and offer 24/7 management, addressing key challenges like security, privacy, and cloud migration complexities.
Cybersecurity
XSOC, one of our Reaktr.ai solutions, is an advanced, AI-driven cybersecurity platform designed to combat a wide range of digital threats. It provides complete visibility and automated threat detection, covering everything from identity management to penetration testing. This unified solution offers clients crucial insights and robust defense strategies, providing strong resilience against evolving cyber threats. Key benefits of XSOC include comprehensive visibility, efficient management through a single platform, and effective risk mitigation, thereby safeguarding critical business assets and providing secure, uninterrupted operations.
ContractX.AI
Navigating contract management demands expertise and a team of legal and paralegal professionals. ContractX.AI leverages Generative AI with Large Language Models (LLMs) to adeptly identify and extract key elements such as attributes, clauses, obligations, and potential risks from any contract. This innovation addresses the challenges faced by Legal Counsels and extensive legal departments in achieving a unified, comprehensive view of contracts, highlighting risk profiles, liabilities, jurisdictional complexities, and other critical dimensions.
AgentX.AI
Conversational AI helps businesses automate various processes like customer service, marketing content generation, sales support, technical support, and many other high-impact organizational processes. This platform brings state-of-the-art Generative AI with LLM to solve these automation problems that translate into substantial business impact for various functions.
Embracing the Power of AI
Reaktr.ai stands out as a prime example of the transformative impact of artificial intelligence in business operations. By co-creating AI solutions with Exela based on business needs, businesses can leverage state-of-the-art AI solutions for enhanced security, data management, and operational efficiency. Reaktr.ai not only provides AI solutions but also redefines the way businesses adapt to and thrive in the digital landscape, showcasing AI’s vast potential in driving business innovation and growth.
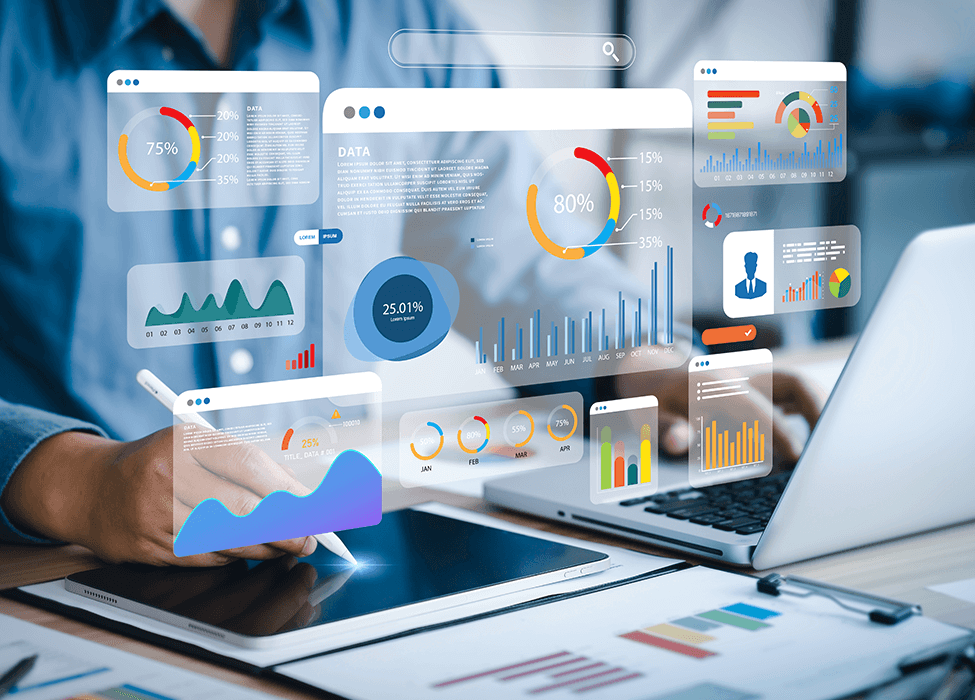
AI in Business: Charting the Path Forward
The journey of integrating artificial intelligence in business operations is an ongoing process of learning, adapting, and innovating. As a business leader, embracing AI can unlock immense potential for your organization, leading to unparalleled efficiency and growth. Remember, the key to successful AI implementation lies in strategic planning, continuous learning, and staying abreast of technological advancements. Embrace the AI revolution with Exela’s Reaktr.ai, and lead your business towards a smarter, more efficient future.