In our data-drenched world, distinguishing between data science and statistics isn’t always clear. It’s no wonder people are scratching their heads in confusion, given that both data science and statistics share many similarities. However, these disciplines, while rooted in the quest for knowledge through data, take different paths toward their goal of insights and solutions. This blog aims to clarify these differences and explore how each field contributes uniquely to businesses.
Data Science vs Statistics: Understanding the Foundations
First, we need to look at the foundations to determine the difference between data science in business and statistics in business. Statistics, a traditional pillar of mathematical science, is primarily focused on collecting data, performing statistical analysis, and drawing conclusions based on sample data. The essence of statistics is to provide a predictive model that can be generalized to a larger population, using robust statistical methods to ensure validity and accuracy.
Example: In an e-commerce setting, statisticians might analyze customer purchase data to determine if there's a significant increase in sales following specific marketing campaigns or seasonal promotions. By conducting hypothesis tests and analyzing variance (ANOVA), statisticians can identify which factors most strongly influence purchase decisions. This insight helps businesses tailor their inventory and marketing strategies to better align with consumer behavior.
Conversely, data science is an interdisciplinary field that blends computer science, statistics, and machine learning to analyze and interpret complex data sets. Data scientists and statisticians often work together, utilizing advanced programming languages such as Python, R, and Java to develop sophisticated models that can predict trends and automate decision-making processes.
Example: On the data science front, the approach involves a more dynamic interaction with data. In the same e-commerce scenario, data scientists would take the analysis further by employing machine learning techniques to not only understand past behaviors but also predict future actions of customers. For instance, by analyzing browsing and purchase histories, data scientists can build machine learning models that predict what products a customer is likely to be interested in next. This level of predictive modeling enables personalized recommendations for each customer during their website visit, potentially enhancing the user experience and increasing sales.
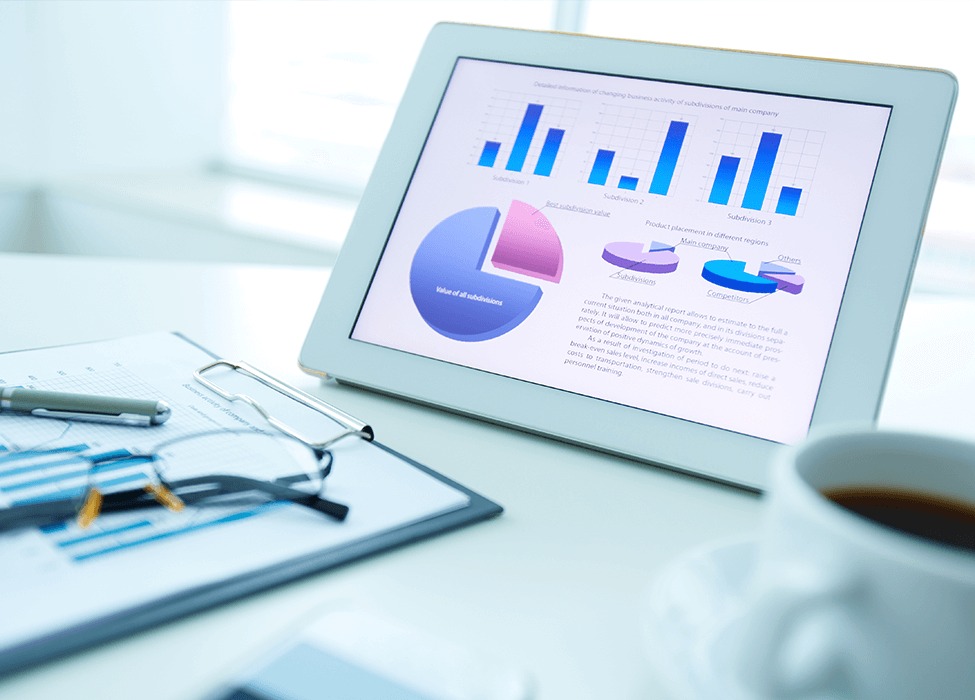
Tools and Techniques at Play
Another major difference between data science and statistics is the tools they use. Statisticians typically rely on statistical tools like SPSS, SAS, and R, which are tailored for rigorous statistical tests and data analysis. These tools are integral in hypothesis testing and regression analysis, staples in the statistician's toolkit.
Example: In the context of a streaming service, statisticians might use tools like R to analyze viewer ratings and feedback for different shows to assess their popularity and satisfaction levels. By employing statistical methods such as ANOVA, they can compare the average viewer ratings across various genres or time slots to determine if the differences in viewer satisfaction are statistically significant. This analysis helps the streaming service understand which types of content are performing well and which are not, guiding decisions on content curation and scheduling.
Data scientists, on the other hand, use a variety of programming languages and frameworks, including machine learning libraries. These are not only crucial for developing machine learning models but also for handling big data—datasets so large and complex that traditional data processing applications are inadequate.
Example: Data scientists at the streaming service take a broader approach by using advanced programming languages and machine learning tools. They develop complex models that not only analyze current viewer preferences but also predict future viewing habits based on comprehensive data sets, including viewer engagement data, browsing history, and social media sentiment. For instance, a machine learning model could predict the potential popularity of new shows based on similarities to highly rated ones. This predictive capability enables the streaming service to dynamically tailor its promotions and recommendations to individual users, potentially enhancing viewer engagement and retention.
Approaches to Problem-Solving
The problem-solving approaches in statistics and data science also differ markedly. Statistics uses a deductive approach, starting with a theory and using data to test this hypothesis. This method is fundamental in ensuring the reliability and scientific rigor of statistical conclusions.
Example: At a traffic management center, statisticians might hypothesize that traffic congestion increases significantly during public events or rush hours. To test this, they collect traffic flow data from various sensors across the city during different times, including event days and normal days. By applying statistical methods such as regression analysis, they can analyze if the increase in vehicles during these specific times statistically correlates with higher congestion levels. This information helps traffic managers to plan better traffic routing or to schedule maintenance at times that would minimally impact traffic flow.
Data science adopts an inductive approach, particularly when it involves artificial intelligence and machine learning. Data scientists build models based on the data they collect, continuously refining them as more data becomes available. This method is particularly effective in environments where the problems are not well-defined or are subject to rapid changes.
Example: Without starting from a specific hypothesis, data scientists employ algorithms to detect patterns and anomalies in traffic data collected from sensors and cameras. They might use data mining techniques to identify unexpected congestion points and then apply predictive analytics to forecast future congestion scenarios. These forecasts can be used to dynamically adjust traffic signals and signs to alleviate expected congestion, optimizing traffic flow in real time based on current traffic conditions and historical data patterns.
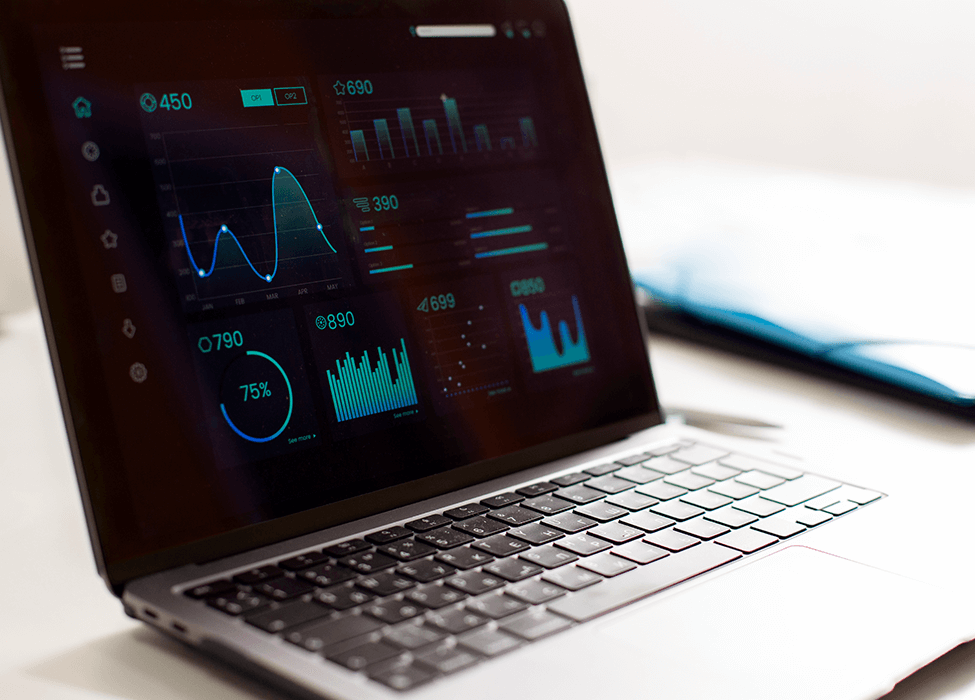
Bridging Theory and Application
While data scientists and statisticians often have different focuses, the two disciplines are not in competition but rather complement each other. Effective data science cannot function without the foundational theories of statistics, and modern statistics has grown to incorporate the computational power and techniques of data science.
Statistical principles are critical in validating and refining predictive models in data science, ensuring that these models are not just powerful but also accurate and trustworthy. Meanwhile, the tools and methodologies developed in data science have expanded the scope of statistical analysis, allowing statisticians to tackle problems involving big data and complex algorithms more effectively.
Example of Statistics and Data Science Integration: In financial services, a team comprising both data scientists and statisticians might collaborate to model credit risk. Statisticians ensure the model adheres to regulatory frameworks by applying traditional statistical tests and confidence intervals to assess model reliability and bias. Meanwhile, data scientists implement advanced machine learning techniques to refine the predictive accuracy of the model, using historical loan performance data. This collaboration ensures that the model not only complies with financial regulations but also efficiently predicts loan defaults, blending statistical rigor with computational innovation.
The Synergy of Data Science and Statistics
The intersection of computer science and statistics through data science marks a dynamic frontier for explorative analysis and predictive modeling. Understanding the unique strengths and methods of data science and statistics is essential for anyone looking to leverage data in making informed decisions. As the volume of data continues to grow, the collaboration between data scientists and statisticians will undoubtedly be central to advancing the frontiers of knowledge, making sense of big data and solving complex problems in innovative ways.
By appreciating both the rigorous testing of statistics and the innovative algorithms of data science, professionals and organizations can harness the full potential of their data, leading to smarter strategies and better outcomes in a data-driven age.
Want to discover how data science solutions can help your business thrive? Contact us today!